Introduction: The Arc of Transformation
As brands began to scale, selling across multiple channels became more than a growth strategy, it became a necessity to meet customers where they are. From marketplaces like Amazon, Walmart, and eBay, to branded storefronts on Shopify, physical retail shelves, and even emerging social platforms like Pinterest, WhatsApp, and TikTok, commerce spread rapidly across touchpoints.
This fragmentation created new operational challenges. Traditional warehouse management systems (WMS) were never designed to handle the complexity of real-time inventory visibility, order orchestration, and fulfillment across such a wide array of sales channels. What brands needed instead was a centralized Fulfillment Operating System (FOS) - a platform that could serve as the connective tissue between every source of demand and every unit of inventory.
At Hopstack, we recognized this need early and built our platform to unify the core pillars of fulfillment - orders, inventory, and workflows across every channel. This solved a critical pain point: it eliminated discrepancies, removed information silos, and gave brands the confidence to scale across channels without breaking their backend.
But just as omnichannel orchestration once became essential, we now stand at the edge of the next shift, one driven not by channel complexity, but by the limitations of human-led operations in a world of increasing scale, speed, and unpredictability.
The future of fulfillment isn’t just omnichannel. It’s autonomous.
The Omnichannel Era - A Necessary Step Forward
To understand where fulfillment is headed, we need to understand where it came from.
The First Wave - From Physical Retail to E-Commerce
Traditionally, commerce revolved around brick-and-mortar retail. Customers walked into physical stores, browsed aisles, and made purchases in person. In this model, warehouses operated primarily as storage and distribution hubs, designed to ship large quantities in pallets and cases of goods to retail shelves or big-box retailers. The systems powering these warehouses, including legacy WMS solutions like SAP, Oracle, and Manhattan, were built to orchestrate this exact flow: bulk replenishment from warehouse to store.
For decades, this model served global commerce well. Large enterprises, especially CPG giants and retailers, structured their supply chains around it. But by the late 2000s and through the 2010s, cracks began to appear, not in the model’s reliability, but in its relevance.
As e-commerce started to rise, driven initially by marketplaces like Amazon and eBay, a new customer behavior emerged. Consumers no longer relied solely on stores, they began to buy online, expecting items to arrive at their doorstep within days, if not hours. This wasn’t just a new channel; it was a new way of thinking about commerce. And as Amazon and others pushed the boundaries with next-day and same-day delivery, customer expectations fundamentally changed.
The first wave of e-commerce was largely marketplace-driven, dominated by the likes of Amazon and eBay. But the second wave - the DTC (direct-to-consumer) revolution saw brands building their own digital storefronts using platforms like Shopify, BigCommerce, and WooCommerce. They also began experimenting with social selling through TikTok and Instagram. Commerce was no longer confined to a “place” - it became channel-fluid.
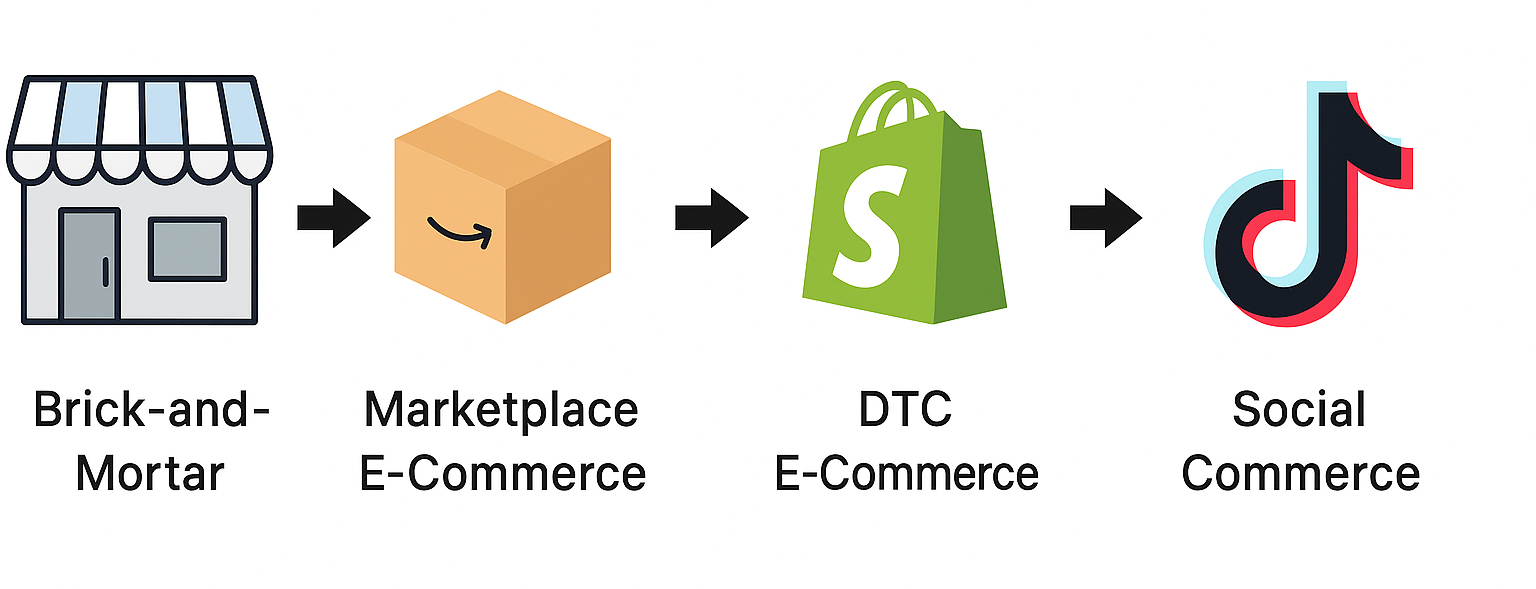
Rise of Omnichannel - The Great Convergence.
Then came the COVID-19 pandemic, which acted as an accelerant. Brands, whether born online or offline, were forced to scale across channels to survive. For digital-first brands, scaling often meant expanding into physical retail: opening stores, getting onto retail shelves, or partnering with wholesale distributors. Warby Parker is a classic example that began as a DTC eyewear startup and eventually opened hundreds of physical stores to reach new customers.
At the same time, traditional brands and enterprises like Unilever, Kraft Heinz, P&G realized they could no longer rely solely on retail partnerships. They needed to go direct to consumers, build their own e-commerce presence, and tap into new revenue streams. Suddenly, companies that were once purely wholesale or retail-first were setting up Shopify storefronts, listing on Amazon, and launching personalized fulfillment experiences.
This convergence meant that every brand whether digital-native or retail-born had to become omnichannel. And that’s where the real challenge began.
Most legacy WMS platforms were built for the pre-ecommerce, bulk-shipping era. They excelled at orchestrating B2B flows but lacked the granularity and speed needed for ecommerce fulfillment. On the flip side, modern cloud-based ecom-first WMS platforms emerged in the 2010s, built specifically for online fulfillment. These worked well for early-stage DTC brands, but struggled with B2B and wholesale workflows once those brands scaled. Neither category of software could handle both worlds effectively.
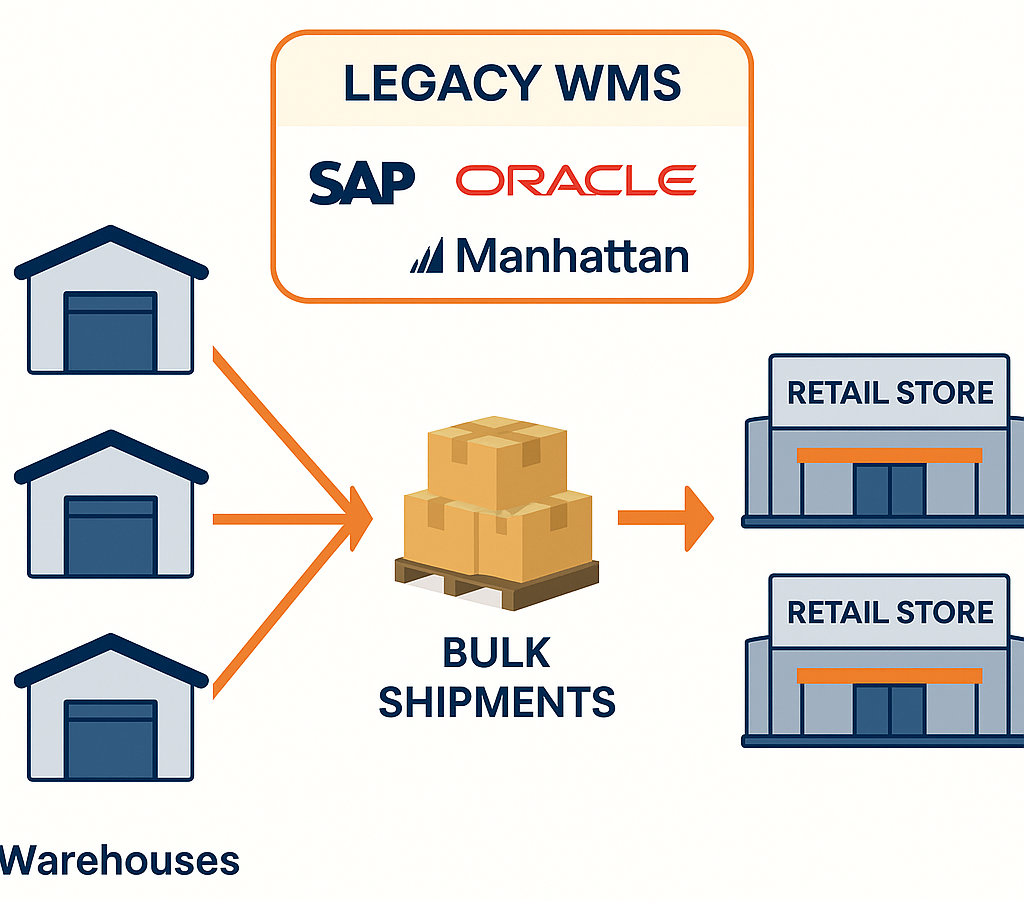
This created a critical white space: the need for an Omni-channel Fulfillment Operating System that could operate seamlessly across channels - ecommerce, wholesale, marketplace, and retail. A system that could consolidate inventory visibility, synchronize order flows, and streamline fulfillment regardless of where the order originated or how it needed to be shipped.
That’s the origin story of omnichannel fulfillment as a necessary evolution in response to shifting consumer preferences. The modern brand, and the modern 3PL could no longer function in silos. They needed one unified platform to serve every customer, every order, and every channel without compromise.
The New Bottlenecks in Omni-Channel Fulfillment
Omnichannel fulfillment platforms brought structure to a previously fragmented landscape. They unified inventory across sales channels, streamlined order flows, and gave operations teams a single pane of glass. But even with that orchestration in place, a new layer of complexity has emerged - one that orchestration alone cannot solve.
These are not challenges of visibility or control. They are challenges of decision-making under scale, responding to dynamic events, and operating with fragmented tribal knowledge across fast-changing workflows. Whether it's high-end retail, FBA prep, or cross-border commerce, the human planner and increasingly, the rule engine has become the bottleneck.
Manual Planning and the Limits of Human Bandwidth
At a luxury goods 3PL, the warehouse manager routinely arrives at 4:00 a.m. during peak season to manually sequence thousands of daily orders. This isn't just about pick-release. It's a meticulous 18-step checklist where every order is classified based on customer tier, promised delivery date, customs documentation requirements for international orders, carrier pickup schedules, and more.
Some of these variables are captured as data points in the WMS, while others live only in the planner’s head. As long as volumes were modest, this approach worked. But during flash sales or holiday spikes, when the order volume jumps 10x, the only available options are to either throw more people at the problem or hard-code the decision logic into custom scripts or a rule engine.
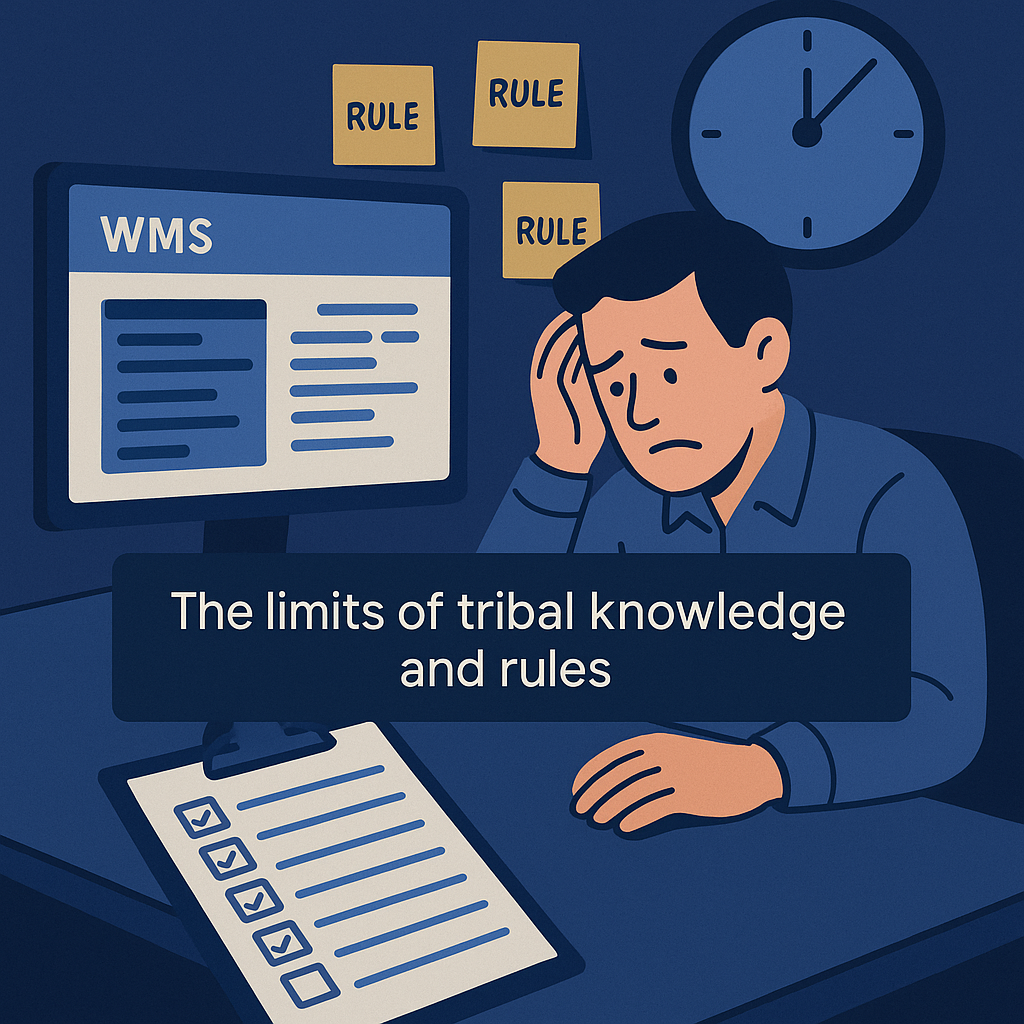
Rule Engines: Static Solutions in a Dynamic World
Codifying decision logic might appear like progress, but rule engines have their own limitations. They are only as good as the assumptions baked into them, and they do not adapt well to live operational shifts.
For example, consider a scenario where one of the primary carriers updates its pickup window mid-day due to network delays, or a key SKU goes out of stock after the first wave of picking. A rule engine, designed to operate on predefined logic, fails to adjust on its own. The decision needs to be made dynamically, on the fly, which neither a rigid ruleset nor a manual planner can handle efficiently without causing delays, mis-shipments, or SLA breaches.
The Cost of Errors: Label Failures and Return Spirals
We’ve seen examples in e-commerce fulfillment where human oversight leads to large-scale returns. In one case, a warehouse had over 400 parcels returned by the carrier in a single day because the printed shipping labels were missing required data fields. The WMS had the correct logic, but the data fed into it was incorrectly input by a floor associate.
These failures don’t just result in return shipping costs. They create a ripple effect: reprocessing inventory, negative customer experience, increased support volumes, and often penalties from marketplaces.
FBA Prep Workflows: Too Many Decisions, Not Enough Automation
The FBA prep flow, in particular, showcases how deeply human-dependent warehouse operations still are.
A 3PL handling shipments for multiple Amazon sellers must constantly:
- Monitor seller inventory levels on Amazon to decide when to create FBA replenishment plans
- Consider thresholds defined in their contract (e.g., auto-ship inventory within 48 hours of receiving it from a supplier, or only after hitting a certain threshold on the Seller Central Account)
- Select between various Amazon placement and transportation options, often splitting shipments across multiple FCs
- Evaluate receiving backlogs at different Amazon FCs to account for delays
- Handle eligibility checks for hazmat products or those with expiry dates, ensuring compliance before the shipment is created
These decisions are not made once. They are repeated every day, for dozens of brands, each with different logic, constraints, and account setups. And most of these workflows are still driven by warehouse managers manually navigating Seller Central and the WMS hoping their decisions match dynamic system conditions.
The Pattern Across Channels
Whether it’s Wholesale Orders, FBA Shipments, or Cross-border commerce, the story is the same:
- The scale and speed of order fulfillment exceed what human planners can manage.
- Rule engines, while better than spreadsheets, are rigid and brittle in dynamic environments.
- Errors compound quickly, leading to financial loss, customer dissatisfaction, and reputational risk.
This is not a failure of software, but a limitation of how current systems think. They require precise inputs, clear configurations, and predictable patterns. But the fulfillment world is none of those things anymore.
The next leap will not be about connecting more systems. It will be about creating systems that can reason, adapt, and respond, not just execute. That’s the foundation on which autonomous fulfillment will be built.
The Future of Fulfillment is Autonomous
Even with omnichannel fulfillment platforms in place, many warehouse operations remain stubbornly manual, especially when it comes to planning, prioritization, and handling exceptions.
From deciding which orders to ship first to determining when and how to replenish Amazon inventory, these decisions are still made by people or baked into rigid rule engines. Rules can encode known logic, but they fall short when real-world complexity, timing, or exceptions come into play.
The next evolution is clear: we need systems that can think, not just follow instructions.
This is the shift from orchestrated to autonomous fulfillment.
Autonomous doesn’t mean science fiction or robot-run warehouses. It means systems that understand context, evaluate trade-offs, anticipate issues, and make decisions like a warehouse planner, but at scale, with consistency, and in real time.
Let’s explore what this looks like in the real world.
Dynamic Outbound Planning based on Live Constraints
In most warehouses today, pick lists are generated at fixed intervals or manually released in large batches. These are often based on filters like carrier, shipping method, or SLA deadline.
An autonomous system doesn’t wait for someone to press “release.” It constantly monitors the floor and generates micro-batches of orders that:
- Share common SKUs or picking zones, reducing walking time
- Match with available labor and equipment
- Prioritize high-value or time-sensitive orders
- Avoid zones currently experiencing congestion or delays
For instance, if a set of orders has overlapping SKUs stored in a quiet zone where a picker just became available, the system may create and assign that batch instantly, optimizing throughput without human intervention.
FBA Shipment Planning with Constraint Awareness
For 3PLs handling Amazon FBA prep, sending replenishment shipments isn’t as simple as "when inventory runs low." Planners must consider:
- Inventory thresholds for each seller on Amazon
- Whether the 3PL contract allows for just-in-time shipping or mandates scheduled dispatches
- Receiving backlog at different Amazon fulfillment centers
- The placement group options Amazon suggests (which may split shipments)
- Carrier performance on each route
An autonomous system would:
- Monitor Amazon inventory levels and seller account status
- Forecast when inventory is likely to run out
- Weigh contract constraints and FC backlogs
- Recommend the optimal shipment time, FC, and carrier
- Draft the plan and surface it to the operator for approval
Instead of toggling between Seller Central, spreadsheets, and the WMS, the warehouse manager becomes a reviewer, not the sole driver.
Exception-Based Decision-Making When Carrier Cutoffs Shift
Let’s say a preferred carrier suddenly moves up their pickup by 90 minutes due to regional weather. In a traditional WMS, that information requires someone to manually re-sequence orders or pause certain waves. Often, it's caught too late, and some orders miss their promised delivery date.
An autonomous system:
- Detects the carrier update via API or email ingestion
- Scans open orders to identify those impacted
- Re-sequence picking for those orders or switch the carrier based on available rate cards
- Notifies the planner only if the alternate carrier increases cost or changes SLA
This turns what would have been a rush-hour scramble into a calm, informed adjustment.

Intelligent Replenishment and Slotting During Peak
In high-SKU, high-throughput environments (such as beauty or consumer electronics), peak season introduces volatility that disrupts slotting and replenishment cycles.
Traditionally, warehouse staff rely on static ABC classification or past averages to determine SKU placement and replenishment frequency. But these patterns break down when demand spikes unexpectedly.
An autonomous system:
- Continuously monitors pick velocity and forecasted demand
- Flags SKUs that are being under-picked or over-picked relative to their slot location
- Suggests pre-emptive re-slotting or shortens replenishment thresholds
- Takes seasonal trends into account (e.g., moving gift sets closer to pack zones in November)
Rather than waiting for problems to show up in throughput reports, the system adjusts in advance.
Real-Time Reclassification of Cross-Border Shipments post 321 suspension
When the U.S. government suspended Section 321 recently, removing duty-free status for sub-$800 shipments, many cross-border 3PLs had to manually reclassify thousands of orders for customs compliance.
In most WMS setups, this meant updating rule logic, re-tagging orders, and regenerating shipping labels, while risking rejections from carriers or customs authorities.
An autonomous system:
- Detects customs flag changes or duty policy updates via connected systems or feedback loops.
- Reclassifies affected shipments based on new rules
- Reassigns carriers or holds the order if new documentation is required
- Triggers alerts only for shipments that cannot be automatically rerouted
This level of agility, responding to regulatory changes within minutes instead of days, simply isn’t feasible without autonomous systems.
Proactive Exception Handling in Multi-Warehouse Order Routing
For brands or 3PLs operating multiple fulfillment centers, routing an order is typically rule-based:
- Fulfill from the warehouse closest to the customer with available stock.
But what if:
- The nearest FC is short-staffed today?
- The carrier SLA is impacted by a storm in that region?
- Another FC has slow-moving stock that needs liquidation?
An autonomous system:
- Monitors real-time labor availability and SLA risk by location
- Understands the margin impact of shipping from each FC based on product velocity and cost
- Re-routes an order to a non-default FC to balance load, clear aged stock, or protect against service disruption
- Surfaces a justification with each decision (e.g., “SLA risk in FC1; rerouting to FC2 ensures on-time delivery”)
Instead of pre-built heuristics, the system exercises real-time judgment based on operational health, something rule-based logic cannot match.
Autonomous Billing Reconciliation Between 3PLs and Brands
Billing between 3PLs and their brand clients is notoriously complex. Charges are based on diverse service-level agreements: per-pick fees, storage by volume or SKU count, special handling surcharges, packaging materials, and FBA prep activities. These are typically tracked across disparate systems including WMS, spreadsheets, manual inputs, and compiled into invoices at the end of a billing cycle.
Reconciliation often becomes a multi-day back-and-forth:
- Brands dispute charges they don’t understand
- 3PLs manually audit order histories and activity logs to justify each line item
- Mismatches in interpretation (e.g., when does storage start counting? What qualifies as “special handling”?) create distrust
An autonomous system reduces friction by introducing real-time, rules-aware billing intelligence:
- Every chargeable event (pick, pack, storage, prep, return) is logged at the moment it happens with relevant metadata (order ID, SKU, operator, timestamp)
- The system auto-tags events with the correct rate card reference, based on the contract with that specific brand
- At the end of the cycle, the invoice is auto-generated with full transparency, each line item backed by traceable activity and justification
- Disputes are minimized because the why and when of each charge is evident, not hidden behind a black-box rate calculation
From Orchestration to Judgment
Omnichannel fulfillment solved the problem of fragmentation. It connected sales channels, streamlined data flows, and brought structure to a chaotic stack. Autonomous fulfillment will teach them how to judge.
Judgment is the missing piece today: the ability to know which orders matter more, when it’s worth waiting, what tradeoffs are acceptable, and how to act under uncertainty.
Humans are good at this, but not 10,000 times a day. And not with data flowing in from 12 sales channels, 6 carrier APIs, 3 fulfillment centers, and thousands of SKUs with seasonality and shelf-life considerations.
That’s where autonomy matters. Not because it replaces the human, but it carries the cognitive load at scale, especially in moments of peak pressure, high complexity, or unpredictable change.
The Path Ahead at Hopstack
Over the last decade, fulfillment platforms evolved to meet the demands of omnichannel commerce - unifying data, orders, and workflows across fragmented channels. At Hopstack, we were early to recognize this shift and built our platform with orchestration at its core. But orchestration is no longer enough.
The next era of fulfillment will be shaped by autonomy, not in the sense of robots replacing people, but in the emergence of intelligent systems that can reason, prioritize, and act alongside humans.
We’re entering a world where the warehouse doesn’t wait for a planner to press “release wave.” Where pick lists are not generated at static intervals but are adapted every few minutes based on inventory movement and carrier cutoffs. Where slotting decisions are no longer made during quarterly reviews, but continuously, based on data signals flowing through the system.
This is the future of Autonomous fulfillment:
- One where AI doesn’t just sit behind dashboards but steps into the decision loop
- One where systems don’t just show what’s wrong, but propose what to do next
- One where fulfillment becomes proactive, adaptive, and self-optimizing
At Hopstack, we’re building toward this future - layer by layer, use case by use case. Because the real leap isn’t from omnichannel to autonomous. It’s from instruction-based execution to intent-aware action.
And that leap is already underway.